Robust Statistics applied to location and scale measures: Technical Note.
Abstract
When measurement of a variable is conducted, it is common for estimates of a sample to have a substantial amount of bias. Some factors responsible of this outcome are asymmetry and outliers. In psychology and the social sciences, it is usual to find that most of the commonly used statistics, such as the arithmetic mean and its associated standard error are, at best, imprecise in the task of data summary and inference. To overcome this situation, researchers can make use of robust statistics. Robust statistics provide a series of alternative estimates resistant to the influence of outliers, resulting in more precise information and inferences. The aim of this paper is to describe a group of procedures to calculate measures of location and scale with robust methods using R and IBM SPSS software. First, the different visual and quantitative methods for detecting outliers are reviewed. Then, different alternatives of location measures are reviewed such as the trimmed mean, the winsorized mean and M estimators. Each location measure will be presented with its associated standard error. Last, some scale measures are presented, such as the interquartile range and a modification, called ideal fourths. Conclusions emphasize the thoughtful use of the procedures in relation to the reader possibilities, interests and the theoretical and methodological implications.Downloads
References
Casella, G. & Berger, R. (2002). Statistical Inference (2 ed.). Pacific Grove: Duxbury.
Cohen, J. (1988). Statistical Power Analysis for the Behavioral Sciences. 2nd edition. Academic Press, New York.
Emerson, J. D. & Strenio, J. (1983). Boxplots and Batch comparison. En D. C. Hoaglin, F. Mosteller, & J.W. Tukey (Eds.), Understanding Robust and Exploratory Data Analysis. New York: Wiley.
Fernández, S. F., Sánchez, J. M. C., Córdoba, A., & Largo, A. C. (2002). Estadística descriptiva. España: Esic Editorial.
Field, A. (2013). Discovering statistics using IBM SPSS statistics. London: Sage.
Field A. & Wilcox R. (2017). “Robust statistical methods: A primer for clinical psychology and experimental psychopathology researchers.” Behaviour Research and Therapy, 98, 19 38.
Goodall, C. (1983). M-Estimators of location: An outline of the theory. En D. C. Hoaglin, F. Mosteller, & J.W. Tukey (Eds.), Understanding Robust and Exploratory Data Analysis. New York: Wiley.
Hopkins, K. & Glass, G. (1978) Basic Statistics for the Behavioral Sciences. Englewood Cliffs, New Jersey: Prentice-Hall.
Huber, P. (1981) Robust statistics, New York: John Wiley & Sons, Inc.
Nunnally, J. C., & Bernstein, I. H. (1994). Psychometric theory (3rd ed.). New York, NY: McGraw-Hill, Inc.
Press, W., Teukolsky, S., Vetterling, W. & Flannery, B (2007). Numerical Recipes 3rd Edition: The Art of Scientific Computing (3rd. ed.). Cambridge University Press, USA.
Rosenberg, J. L. & Gasko, M. (1983). Comparing Location Estimators: Trimmed Means, Medians, and Trimean. En D. C. Hoaglin, F. Mosteller, & J.W. Tukey (Eds.), Understanding Robust and Exploratory Data Analysis. New York: Wiley.
Rousseeuw, P. J. (1991). Tutorial to robust statistics. Journal of Chemometrics, 5(1), 1-20.
Ruiz, D. (2020). Distribución de la conducta operante en humanos: desarrollo de preferencia y parámetros de reforzamiento relevantes. Tesis doctoral no publicada. Universidad Nacional Autónoma de México.
Tukey, J. W. (1960). A survey of sampling from contaminated normal distributions. In I. Olkin, S. Ghurye, W. Hoeffding, W. Madow, & H. Mann (Eds.), Contributions to Probability and Statistics. Stanford, CA: Stanford University Press.
Wilcox, R. (2017). Introduction to Robust Estimation & Hypothesis Testing. 4th edition. Elsevier, Amsterdam, The Netherlands.
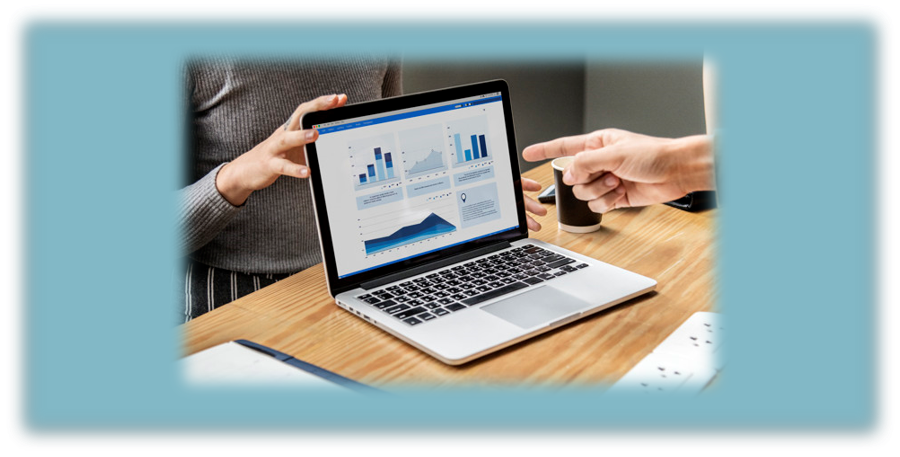
Política de publicación para la Revista Digital Internacional de Psicología y Ciencias Social de acceso abierto
Los autores/as que publiquen en esta revista aceptan los derechos de autor, la Política de privacidad y los Términos de uso de la Revista Digital Internacional de Psicología y Ciencias Social (RDIPyCS), que son en resumen las siguientes:
- Los autores/as conservan los derechos de autor y ceden a la revista el derecho de la primera publicación, con el trabajo registrado con la licencia de atribución de Creative Commons, que permite a terceros utilizar lo publicado siempre que mencionen la autoría del trabajo y a la primera publicación en esta revista.
- Los autores/as pueden realizar otros acuerdos contractuales independientes y adicionales para la distribución no exclusiva de la versión del artículo publicado en esta revista (p. ej., incluirlo en un repositorio institucional o publicarlo en un libro) siempre que indiquen claramente que el trabajo se publicó por primera vez en esta revista.
- El autor o los autores del manuscrito publicado en la Plataforma de la RDIPyCS en cualquiera de sus tipos o modalidades (véase en línea editorial los tipos de estudio) ceden sus derechos sobre el manuscrito publicado y renunciar expresamente, a cualquier acción civil, penal o administrativa en contra de la RDIPyCS y su equipo editorial, respecto de dichos derechos.
- Los autores/as mantienen todos los otros derechos de autor, tales como patente, marcas registradas y los derechos de procesamiento descritos en el manuscrito publicado en la Plataforma de la RDIPyCS en cualquiera de sus tipos o modalidades (veáse en línea editorial los tipos de estudio.
- Los autores/as están de acuerdo que su manuscrito publicado por la RDIyPC sea registrado con la licencia de atribuciones Creative Commons, que permite a terceros utilizar lo publicado siempre que se mencione la autoría del trabajo.
- Los autores/as del manuscrito publicado podrán utilizarlo para fines educativos o de investigación, reproducción y difusión en todas las formas posibles.
- Estos términos de uso no afecta a los derechos morales del autor o los autores sobre el manuscrito publicado.
- Los autores/as aceptan la Política de privacidad de la Revista Digital Internacional de Psicología y Ciencias Social. En caso de desconocerla le recomendamos ir a este vínculo y leala detenidamente, ya que al enviar el manuscrito a dictamen usted la está aceptando.
- Los autores/as aceptan los Términos de uso de la Revista Digital Internacional de Psicología y Ciencias Social. En caso de desconocerlos le recomendamos ir a este vínculo y lealos detenidamente, ya que al enviar el manuscrito a dictamen usted los está aceptando.
- Los autores/as aceptan, en caso de que el manuscrito enviado sea publicado por la RDIPyCS, a que el historial del proceso editorialde dictaminación sea también de libre acceso en Internet en una sección del sitio de la RDIPyCS que contiene:
- La versión original del manuscrito enviado con toda la información anexa que los autores/as enviaron.
- Los archivos con los comentarios que los revisores hicieron al manuscrito.
- El dictamen preliminar que los revisores emitieron acerca de manuscrito.
- La respuesta del autor o los autores a los comentarios de los revisores.
- La calificación que le otorgaron los revisores al manuscrito con base en la evaluación cuantitativa que hicieron, para conocer en detalle las dimensiones de la evaluación cuantitativa que se hace a los manuscritos le recomendamos ir a este vínculo, en el apartado sistema de evaluación de los manuscritos y en la opción modelo de evaluación.
- La figura del perfil del manuscrito que se obtuvo al comparar la calificación otorgada por los revisores en cada una de las dimensiones de la evaluación cuantitativa que se realizó.
- La matriz de acuerdo entre revisores y el índice de acuerdo global.
La Revista Digital Internacional de Psicología y Ciencia Social se adhiere, edita y publica bajo los lineamientos éticos establecidos por COPE (Code of Conduct and Best Practices Guidelines for Journals Editors, COPE), así como sus Principios de Transparencia y Mejores Prácticas en Publicaciones Académicas. https://publicationethics.org/
Revista Digital Internacional de Psicología y Ciencia Social de Arturo Silva Rodríguez está bajo una licencia de Creative Commons Atribución-NoComercial-CompartirIgual 4.0 Internacional.
Creada a partir de la obra en http://cuved.unam.mx/rdipycs/.